7 Classic Foundational Vis Papers You Might not Want to Publicly Confess you Don’t Know
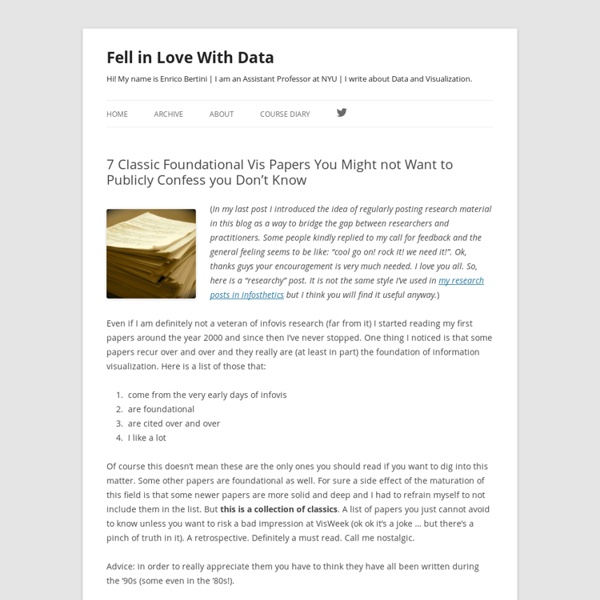
(In my last post I introduced the idea of regularly posting research material in this blog as a way to bridge the gap between researchers and practitioners. Some people kindly replied to my call for feedback and the general feeling seems to be like: “cool go on! rock it! we need it!”. Ok, thanks guys your encouragement is very much needed. I love you all. Even if I am definitely not a veteran of infovis research (far from it) I started reading my first papers around the year 2000 and since then I’ve never stopped. come from the very early days of infovisare foundationalare cited over and overI like a lot Of course this doesn’t mean these are the only ones you should read if you want to dig into this matter. Advice: in order to really appreciate them you have to think they have all been written during the ’90s (some even in the ’80s!). Graphical Perception: Theory, Experimentation, and Application to the Development of Graphical Methods. Please don’t tell me you don’t know this one!
Stephen Few: Information Visualization Research Projects that Would Benefit Practitioners
In a previous blog post titled “Potential Information Visualization Research Projects,” I announced that I would prepare a list of potential research projects that would address actual problems and needs that are faced by data visualization practitioners. So far I’ve prepared an initial 33-project list to seed an ongoing effort, which I’ll do my best to maintain as new ideas emerge and old ideas are actually addressed by researchers. These projects do not appear in any particular order. Some of the projects that appear in the Effectiveness and Efficiency Tests section have been the subject matter of past projects. Please feel free to respond to this blog post or to me directly at any time with suggestions for additional research projects or with information about any projects on this list that are actually in process or already completed. Effectiveness and Efficiency Tests New Solution Designs and Tests Taxonomies and Guidelines Take care,
Information Visualization Research as Pseudo-Science - Perceptual Edge Discussion Forum
Despite the unnecessarily aggressive tone, critiques like this are important for the advancement of science. I am looking forward to the authors' responses and I hope this critique will start an insightful and constructive debate. Although I haven't had the chance to read the paper yet, the part on statistical unreliability seems exaggerated irrespective of the paper's content. The proper choice of sample size does depend on several factors. A few are already mentioned, but the most important of all is effect size. Sample representativeness is also important to consider, but no researcher tries to collect a truly random sample of the entire world's population, including in cognitive sciences where "the scientific method" is used. Power analysis can improve study design, but it is hard to put in practice and is not without problems. No one would question that an ideal study should use power analysis and a large sample size that is representative of a meaningful population.
The Visualization Whiteboard
GitHub - tmcw/perception: collected & summarized research on the effectiveness of visualization techniques
39 studies about human perception in 30 minutes – Medium
bars and pies for proportions Much is said about the relative merits of bars and circles for showing proportions. All five of these studies legitimize the use of pie charts when conveying proportions and some even show their superiority over bar charts. I did not encounter any studies that said we should not use pie charts for showing proportions in all cases. Eells (7) was among the first to publish a paper on this topic in 1926. He also wanted to know more about how circles were processed. Not only did he find that pie charts were read as easily, quickly and accurately as bar charts, but that as the number of components in the chart increased, bars become less efficient encoding the data. He found that 50 percent of people use the outer arc to make proportional judgments, while 25 percent use area, and the other 25 percent use the inner arc or angle. He concluded that we ought to use pie charts, not just for their appeal but because of their scientific accuracy.
Interactive Dynamics for Visual Analysis
Graphics Jeffrey Heer, Stanford University Ben Shneiderman, University of Maryland, College Park The increasing scale and availability of digital data provides an extraordinary resource for informing public policy, scientific discovery, business strategy, and even our personal lives. To get the most out of such data, however, users must be able to make sense of it: to pursue questions, uncover patterns of interest, and identify (and potentially correct) errors. In concert with data-management systems and statistical algorithms, analysis requires contextualized human judgments regarding the domain-specific significance of the clusters, trends, and outliers discovered in data. Visualization provides a powerful means of making sense of data. The goal of this article is to assist designers, researchers, professional analysts, procurement officers, educators, and students in evaluating and creating visual analysis tools. Some visualization system designers have explored alternative approaches.
Related:
Related: