Bayesian Methods for Hackers
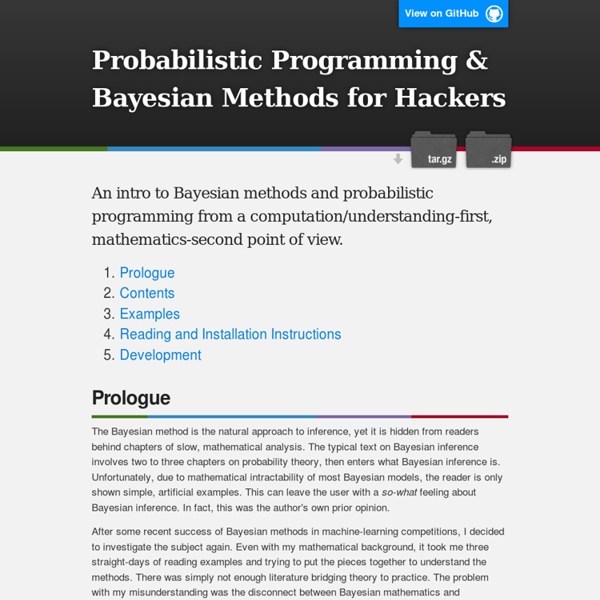
An intro to Bayesian methods and probabilistic programming from a computation/understanding-first, mathematics-second point of view. Prologue The Bayesian method is the natural approach to inference, yet it is hidden from readers behind chapters of slow, mathematical analysis. The typical text on Bayesian inference involves two to three chapters on probability theory, then enters what Bayesian inference is. Unfortunately, due to mathematical intractability of most Bayesian models, the reader is only shown simple, artificial examples. This can leave the user with a so-what feeling about Bayesian inference. After some recent success of Bayesian methods in machine-learning competitions, I decided to investigate the subject again. If Bayesian inference is the destination, then mathematical analysis is a particular path towards it. Bayesian Methods for Hackers is designed as a introduction to Bayesian inference from a computational/understanding-first, and mathematics-second, point of view.
Neural networks and deep learning
The human visual system is one of the wonders of the world. Consider the following sequence of handwritten digits: Most people effortlessly recognize those digits as 504192. That ease is deceptive. The difficulty of visual pattern recognition becomes apparent if you attempt to write a computer program to recognize digits like those above. Neural networks approach the problem in a different way. and then develop a system which can learn from those training examples. In this chapter we'll write a computer program implementing a neural network that learns to recognize handwritten digits. We're focusing on handwriting recognition because it's an excellent prototype problem for learning about neural networks in general. Of course, if the point of the chapter was only to write a computer program to recognize handwritten digits, then the chapter would be much shorter! Perceptrons What is a neural network? So how do perceptrons work? That's all there is to how a perceptron works! is a shorthand.
Related: