Exploratory data analysis
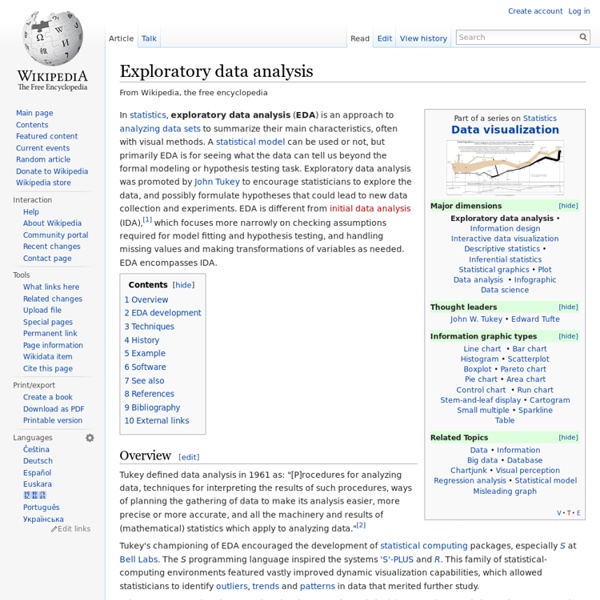
In statistics, exploratory data analysis (EDA) is an approach to analyzing data sets to summarize their main characteristics, often with visual methods. A statistical model can be used or not, but primarily EDA is for seeing what the data can tell us beyond the formal modeling or hypothesis testing task. Exploratory data analysis was promoted by John Tukey to encourage statisticians to explore the data, and possibly formulate hypotheses that could lead to new data collection and experiments. EDA is different from initial data analysis (IDA),[1] which focuses more narrowly on checking assumptions required for model fitting and hypothesis testing, and handling missing values and making transformations of variables as needed. Overview[edit] Exploratory data analysis, robust statistics, nonparametric statistics, and the development of statistical programming languages facilitated statisticians' work on scientific and engineering problems. EDA development[edit] Data science process flowchart
Multilinear principal component analysis
Multilinear principal component analysis (MPCA)[1] is a mathematical procedure that uses multiple orthogonal transformations to convert a set of multidimensional objects into another set of multidimensional objects of lower dimensions. There is one orthogonal (linear) transformation for each dimension (mode): hence multilinear. This transformation aims to capture as high a variance as possible, accounting for as much of the variability in the data as possible, subject to the constraint of mode-wise orthogonality. MPCA is a multilinear extension of principal component analysis (PCA). The major difference is that PCA needs to reshape a multidimensional object into a vector, while MPCA operates directly on multidimensional objects through mode-wise processing. E.g., for 100x100 images, PCA operates on vectors of 10000x1 while MPCA operates on vectors of 100x1 in two modes. MPCA is a basic algorithm for dimension reduction via multilinear subspace learning. The algorithm[edit]
Parallel coordinates
Parallel coordinates is a common way of visualizing high-dimensional geometry and analyzing multivariate data. This visualization is closely related to time series visualization, except that it is applied to data where the axes do not correspond to points in time, and therefore do not have a natural order. Therefore, different axis arrangements may be of interest. History[edit] Parallel coordinates were often said to be invented by Philbert Maurice d'Ocagne (fr) in 1885,[1] but even though the words "Coordonnées parallèles" appear in the book title this work has nothing to do with the visualization techniques of the same name (the book only describes a method of coordinate transformation, see fulltext PDF of the book by clicking the link in the references). Higher dimensions[edit] Adding more dimensions in parallel coordinates (often abbreviated ||-coords or PCs) involves adding more axes. Statistical considerations[edit] Reading[edit] Limitations[edit] Software[edit] See also[edit] Radar chart
Terminology in Data Analytics
As data continue to grow at a faster rate than either population or economic activity, so do organizations' efforts to deal with the data deluge, and use it to capture value. And so do the methods used to analyze data, which creates an expanding set of terms (including some buzzwords) used to describe these methods. This is a field in flux, and different people may have different conceptions of what terms mean. Comments on this page and its "definitions" are welcome. Since many of these terms are subsets of others, or overlapping, the clearest approach is to start with the more specific terms and move to the more general. Predictive modeling: Used when you seek to predict a target (outcome) variable (feature) using records (cases) where the target is known. Predictive analytics: Basically the same thing as predictive modeling, but less specific and technical. Supervised Learning: Another synonym for predictive modeling. Unsupervised Learning: Business intelligence: Data mining: Text mining: 1.
Related:
Related: