Code School - Try R
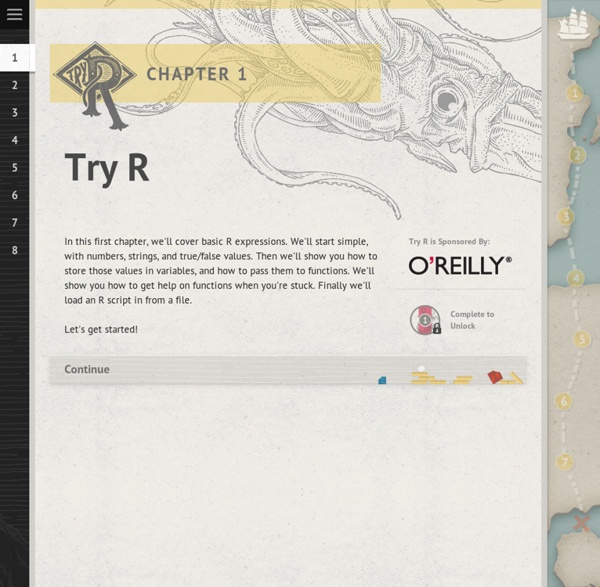
Usability Requirements Template - Super User Friendly
The primary purpose of Usability Requirements is to ensure that the system meets the expectations of its users with regard to its ease-of-use. The expectations of users who have disabilities and employ assistive technologies must also be included. The work product is used to: · Provide baseline guidance to the user interface developers on user interface design. · Establish performance standards for usability evaluations. · Define test scenarios for usability test plans and usability testing. · Document feedback on the usability of the accessibility features and technical accessibility enablement of the system. Note: Accessibility may be conceptually linked to disability; but accessibility also means that people who have older technology, such as modems and earlier versions of browsers, can use the product equally as well as people with the latest technology, such as hardware, software, and Internet connections. Download the Template from the Attachments Section Below
Related:
Related: