UFLDL Tutorial - Ufldl
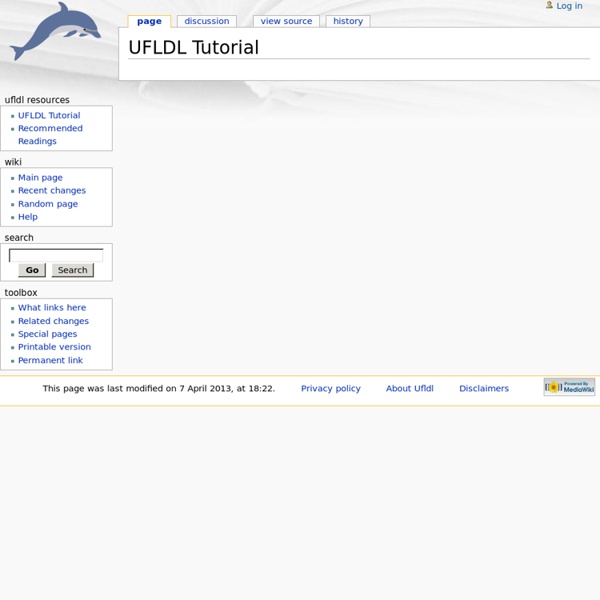
Using large-scale brain simulations for machine learning and A.I.
You probably use machine learning technology dozens of times a day without knowing it—it’s a way of training computers on real-world data, and it enables high-quality speech recognition, practical computer vision, email spam blocking and even self-driving cars. But it’s far from perfect—you’ve probably chuckled at poorly transcribed text, a bad translation or a misidentified image. We believe machine learning could be far more accurate, and that smarter computers could make everyday tasks much easier. So our research team has been working on some new approaches to large-scale machine learning. Today’s machine learning technology takes significant work to adapt to new uses. Fortunately, recent research on self-taught learning (PDF) and deep learning suggests we might be able to rely instead on unlabeled data—such as random images fetched off the web or out of YouTube videos. We’re reporting on these experiments, led by Quoc Le, at ICML this week.
Deep Learning - Community
Related:
Related: